Harnessing AI for Enhanced Fraud Detection in Cryptocurrency
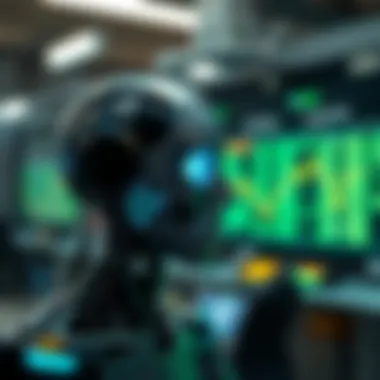
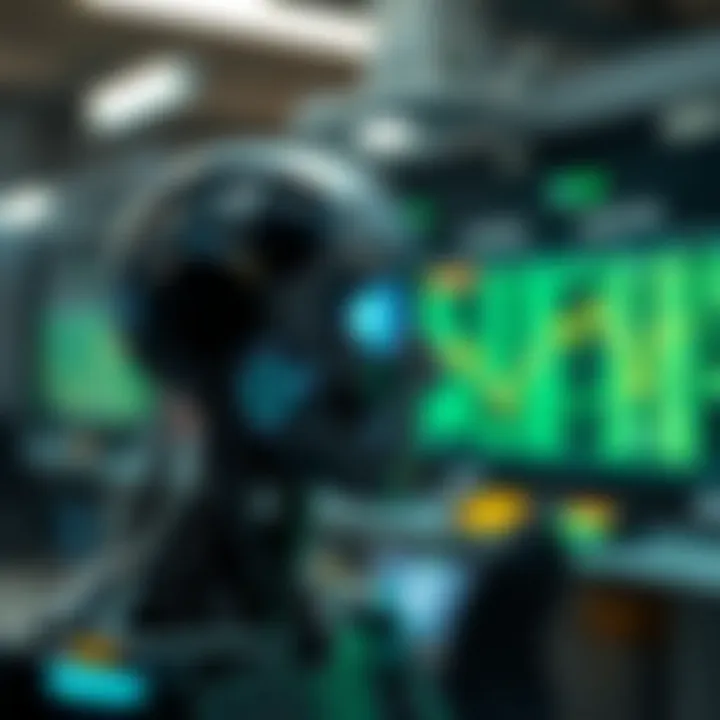
Intro
In recent years, cryptocurrencies have surged in popularity, creating a bustling marketplace rife with both opportunity and risk. As this new financial frontier expands, the vulnerabilities associated with it have come under scrutiny. One major concern has been the prevalence of fraud, casting a shadow over the legitimacy of digital currencies. Consequently, there has been a growing interest in the role of artificial intelligence (AI) in equipping stakeholders with tools to combat fraudulent activities. In this article, we will dissect the intricate relationship between AI and fraud detection in the cryptocurrency realm, elucidating its various techniques, challenges, and future implications.
Understanding Cryptocurrency Fundamentals
Before diving into how AI plays a pivotal role in fraud detection, it's essential to grasp the underlying mechanisms of cryptocurrency and blockchain technology. This foundation will help make sense of the challenges faced in ensuring the security of these digital assets.
What is Cryptocurrency?
Cryptocurrency is a form of digital or virtual currency that uses cryptography for security. Unlike traditional currencies, most cryptocurrencies operate on a technology known as blockchain. The defining feature of cryptocurrencies is their decentralized nature; they are not issued or regulated by a central authority, making them immune to government interference or manipulation. Bitcoin, Ethereum, and Ripple are just a few examples of the many cryptocurrencies available today.
How Blockchain Technology Works
At its core, blockchain technology is a distributed digital ledger that records transactions across many computers in such a way that the registered transactions cannot be altered retroactively. This mechanism not only ensures transparency but also establishes trust among users, as every transaction is publicly verifiable by anyone with access to the network. Each block in the chain contains a list of transactions, a timestamp, and a reference to the previous block, creating an unbreakable link. Here are a few characteristics of blockchain:
- Decentralization: No central authority governs the blockchain, reducing the chances of fraud.
- Transparency: Users can view the entire transaction history, fostering trust.
- Immutability: Once a transaction is recorded, it cannot be altered, which can deter fraudulent behavior.
Despite the advantages of blockchain, the cryptocurrency space isn't free from illicit activities. Unscrupulous individuals exploit the anonymity offered by cryptocurrencies to perpetrate scams and fraud. This is where the integration of AI becomes crucial.
Market Insights and Trends
As we look at the current landscape of the cryptocurrency market, various trends have emerged that highlight the increasing reliance on technology—and AI, in particular. Keeping abreast of these trends is vital for investors, enthusiasts, and educators alike.
Current Market Trends and Analysis
The cryptocurrency market is characterized by rapid fluctuations and evolving innovations. Notably, the adoption of AI in fraud detection has been gaining momentum. Companies are leveraging machine learning algorithms to sift through vast amounts of transactional data, identifying patterns and anomalies that may suggest fraudulent activity.
"AI systems can learn from past data and continuously improve their detection capabilities, making them increasingly effective against sophisticated fraud schemes."
Future Predictions and Investment Strategies
Looking ahead, it’s clear that the interplay between AI and cryptocurrencies will deepen. As algorithms become more sophisticated, they will play an essential role in shaping not only how fraud is detected, but also how investors strategize their investments. Emerging patterns and technologies may dictate new approaches to securing digital assets, combining the best of both realms.
As stakeholders continue to navigate this evolving landscape, understanding the integration of AI within fraud detection will empower them to make safer investment choices and contribute to a more security-conscious cryptocurrency market.
Prolusion to AI in Fraud Detection
In the ever-evolving landscape of cryptocurrency, where digital currencies are the norm, the threat of fraud has also escalated. As more individuals and institutions embrace the opportunities presented by blockchain technology, the need to safeguard these assets grows paramount. This is where artificial intelligence (AI) steps in. AI technologies offer groundbreaking solutions in the realm of fraud detection, making it increasingly feasible to identify and mitigate fraudulent activities before they escalate.
Understanding the role of AI in detecting fraud is crucial for investors, developers, and analysts alike. The primary aim of this integration is to enhance the security of transactions and protect users from potential loss. By diving deep into various AI methods, stakeholders can glean insights into how systems are crafted to recognize the subtle but critical signals of fraud.
Defining Fraud in Cryptocurrency
Fraud in the cryptocurrency space can take numerous forms. Unlike traditional financial fraud, which often encompasses check tampering, credit card schemes, or Ponzi schemes, cryptocurrency fraud often presents itself in more nuanced ways. Examples include phishing scams, where cybercriminals impersonate trusted entities to extract sensitive information from unsuspecting users, and pump-and-dump schemes, where the price of a cryptocurrency is artificially inflated to attract investment before the fraudsters liquidate their holdings.
In essence, fraud in cryptocurrency involves deceiving individuals or organizations with the intent to secure dishonest gains. This behavior is often facilitated by the anonymity and lack of regulation inherent in the cryptocurrency ecosystem, making it harder to trace illicit activities.
The Importance of Detection Systems
Detection systems serve as the first line of defense against fraudulent activities in the crypto world. As digital currencies are transacted at lightning speed, a robust detection system must be in place to monitor activities effectively. These systems are designed to analyze transaction patterns, flagging anomalies that could indicate fraudulent behavior.
The integration of AI into these systems enhances their capabilities significantly. Traditional detection methods might be somewhat effective, but they often fall short in recognizing sophisticated fraud techniques that evolve rapidly. AI algorithms learn from vast amounts of transaction data, honing their ability to identify suspicious behaviors that a human analyst might overlook.
Key benefits of AI in detection systems include:
- Increased Speed: AI can process transactions in real-time, allowing for swift intervention in potentially fraudulent activity.
- Improved Accuracy: Machine learning models can adapt to new fraud patterns, becoming more proficient in detecting anomalies over time.
- Scalability: With the increasing adoption of cryptocurrencies, AI systems can easily scale to accommodate burgeoning databases without losing performance.
In summary, as fraud tactics grow increasingly sophisticated, the critical nature of implementing advanced detection systems becomes glaringly evident. By leveraging AI, stakeholders in the cryptocurrency sphere can establish a crucial defense against fraud.
Understanding Fraud Techniques in the Crypto Space
Understanding the various fraud techniques in the crypto space plays a crucial role in grasping how artificial intelligence can effectively combat these issues. Cryptocurrency's decentralized nature and its rapid evolution have created a fertile ground for fraudulent activities. By recognizing the different types of scams and schemes prevalent in this landscape, stakeholders—from investors to developers—can better appreciate the need for robust fraud detection systems powered by AI.
The significance of this understanding lies not just in identifying threats, but in enhancing overall security within the cryptocurrency ecosystem. With new fraud techniques emerging regularly, it's vital to remain vigilant. This section aims to unravel these fraudulent practices and highlight their implications in the cryptocurrency domain.
Common Types of Cryptocurrency Fraud
Cryptocurrency is a double-edged sword; it has democratized finance, yet it has also birthed numerous fraudulent practices that prey on the uninformed. Some prevalent types include:
- Phishing Scams: Attackers trick users into revealing sensitive information, like private keys or passwords, by mimicking legitimate sites. This technique can persistently plague users, especially if they’re not cautious about the URLs they visit.
- Ponzi Schemes: These schemes rely on new investors to pay returns to older investors, creating an illusion of a profitable business. Once the flow of new investors slows, the scheme collapses, leaving many at a loss.
- Rug Pulls: Common in decentralized finance (DeFi) projects, rug pulls occur when developers abandon the project, taking all the investors' funds with them. Typically, this happens after a token is pumped to attract investors.
- Initial Coin Offering (ICO) Scams: Fake ICOs can solicit funds without any real plan or product. Unsuspecting investors contribute money in exchange for tokens, only for the creators to disappear, often referred to as a 'cash grab.'
Recognizing these types boosts awareness and can drive the adoption of AI solutions that effectively trace and flag suspicious activities.
Emerging Fraud Patterns
As fraudsters become increasingly sophisticated, so do their methods. New patterns are emerging that blend traditional schemes with innovative technology. For instance:
- Deepfake Technology: Fraudsters are now utilizing artificial intelligence to create deceptive videos or audios impersonating credible individuals, like company ceos or influencers, to manipulate investments.
- Decentralized Exchanges (DEX) Exploits: As DEXs rise in popularity, so do the exploits tied to their protocols. Attackers can identify weaknesses in smart contracts, allowing them to siphon funds.
- Social Engineering Tactics: Modern scams often involve a combination of social manipulation along with technological tools, targeting victims emotionally or psychologically to gain trust and access to funds.
Staying ahead of these emerging patterns is complex and demands the urgency of continued education and awareness. The proactive integration of AI into fraud detection systems becomes paramount here to adapt to ever-evolving threats.
In summary, understanding fraud techniques within the cryptocurrency space is essential for building preventative measures. AI can enhance detection capabilities by learning from these established trends and emerging patterns, ultimately shielding the crypto landscape from malign influences.
The Emergence of AI Technologies
The world of cryptocurrency is evolving at an unprecedented pace, and with that evolution comes the responsibility of safeguarding digital assets. As fraud becomes increasingly sophisticated, the necessity for advanced solutions in detection and prevention is paramount. This is where artificial intelligence (AI) steps into the spotlight. The incorporation of AI technologies in fraud detection systems not only streamlines processes but also brings about a level of accuracy and efficiency previously unattainable. Understanding the elements driving this emergence offers critical insights into how AI transforms the landscape of cryptocurrency security.
Machine Learning Fundamentals
When discussing AI in the context of fraud detection, it’s nearly impossible to sidestep machine learning. This subset of AI enables systems to learn from data patterns without being explicitly programmed. At its core, machine learning relies on algorithms that improve over time as they are exposed to more data.
- Supervised Learning: Here, the machine learns from labeled datasets, which means it’s fed examples of fraudulent and non-fraudulent transactions. For instance, a model trained on past transaction data can identify what signals indicate fraud, from unusual patterns to behaviors outside typical spending habits.
- Unsupervised Learning: Unlike its counterpart, unsupervised learning deals with unlabeled data. This can be particularly useful in spotting new fraud patterns that haven’t been previously recorded. A system could categorize transactions based on similarities, flagging those that deviate from normal behavior without prior knowledge of fraudulent traits.
Machine learning models can be tailored for specific applications, enabling tailored solutions for distinct fraud risks inherent in crypto trading. The beauty lies in their capacity to adapt continuously, refining their predictions as they process more information.
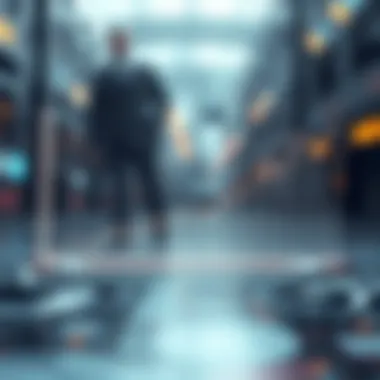
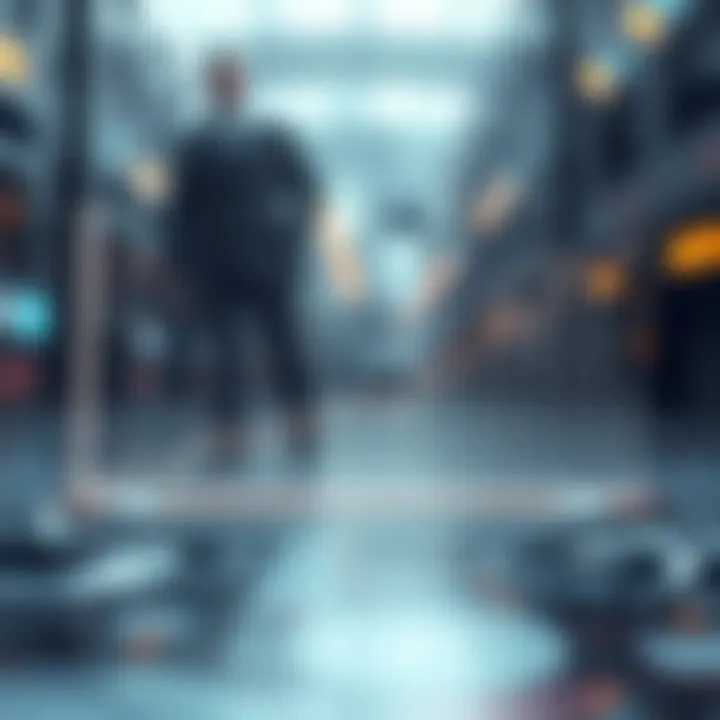
Predictive Analytics in Action
Predictive analytics is where the rubber hits the road. Utilizing historical data, predictive algorithms forecast future outcomes, an invaluable resource for fraud detection. In the cryptocurrency realm, this capability offers a strategic edge.
- Risk Scoring: By analyzing past fraudulent activity, systems can assign a risk score to transactions in real-time. For example, if a transaction appears from an unrecognizable wallet address and involves an unusually large sum, it could automatically trigger alerts or hold until further verification.
- Trend Forecasting: With predictive analytics, stakeholders can identify emerging trends in fraud techniques. For instance, if an uptick in social engineering scams targeting new investors is detected, organizations can proactively take measures to educate their client base about these risks.
AI-driven predictive tools empower stakeholders to stay ahead of fraud criminals. They don’t just react to threats; they anticipate them, making prevention a more achievable objective in the volatile world of cryptocurrency.
Overall, as AI technologies become more integrated into fraud detection systems, they provide a pathway toward more secure and resilient environments. Investors, analysts, and developers must stay cognizant of these trends and engage in continuous education to leverage the benefits these innovations offer.
For more information on the significant role of AI in financial security, visit Wikipedia or explore industry insights at Reddit.
Implementing AI in Fraud Detection Systems
The integration of AI into fraud detection systems marks a crucial evolution in the combat against fraudulent activities within the cryptocurrency sector. It's no longer sufficient to rely solely on traditional methods, where repetitive tasks consume resources and human oversight sometimes falters under the weight of vast data. By employing AI solutions, stakeholders can significantly bolster their defenses, streamline operations, and ultimately protect their assets more effectively.
Data Collection and Preparation
The first step in crafting an AI-powered fraud detection system is robust data collection and preparation. This phase is akin to laying a strong foundation for a building. If the foundation isn't solid, the entire structure may crumble. In the world of cryptocurrencies, data serves as the bedrock upon which AI models are constructed.
Key aspects of data collection include:
- Diverse Data Sources: Fraud detection relies on aggregating data from various sources. This could include transaction records, user behavior analytics, and even external data from social media platforms, like Facebook or Reddit, where discussions about scams often surface.
- Quality Over Quantity: It's vital to prioritize high-quality, relevant data over sheer volume. Irrelevant data can skew model predictions, leading to inaccurate results. It's better to have a smaller dataset that accurately represents the fraud landscape than a massive one full of noise.
- Normalization: Once collected, data needs to undergo a normalization process. This ensures consistency in formats and scales across different data types. For instance, if transaction amounts are logged in various currencies, they need to be converted to a common base currency.
Through diligent data preparation, systems can harness cleansing techniques that sift out anomalies or errors that can mislead AI models during their training phases. Getting this part right is non-negotiable for the success of any subsequent AI applications.
Model Training and Validation
Following data preparation, model training and validation occur. This is where theoretical algorithms start to transform into actionable insights.
AI models learn by identifying patterns within the training data—a critical process for recognizing fraud.
Important considerations in this phase include:
- Algorithm Selection: The choice of algorithms is paramount. Some models, like neural networks, are good at recognizing complex patterns, while others, like decision trees, are easier to interpret. Depending on the specific needs of the fraud detection system, a hybrid approach might even be beneficial, blending multiple algorithms to enhance detection accuracy.
- Overfitting vs. Underfitting: This is the balancing act every data scientist must master. Overfitting occurs when the model learns the training data too well, failing to generalize to unseen cases. Underfitting, on the other hand, happens when the model is too simplistic, missing the nuanced indicators of fraud that real-world data often presents.
- Validation Techniques: Techniques like cross-validation come into play to evaluate how the model performs on different subsets of the dataset. This helps ensure the model is not just a one-hit wonder.
- Performance Metrics: Metrics such as precision, recall, and F1-score assist in gauging the effectiveness of the model, facilitating a more informed understanding of its strengths and weaknesses.
By diligently training and validating models, stakeholders can reinforce the system's ability to detect various fraud schemes with a high degree of accuracy. The time invested in this phase pays off in the long run, as it forms the backbone of a trustworthy fraud detection system.
In sum, implementing AI in fraud detection systems is not merely about adopting new technology; it involves a comprehensive approach that weaves together data collection, preparation, model training, and validation to create a resilient framework capable of adapting to the ever-evolving landscape of cryptocurrency fraud.
Benefits of AI-Led Fraud Detection
The rise of artificial intelligence has brought about transformative changes in various sectors, and fraud detection in cryptocurrency is no exception. Implementing AI technologies offers several benefits, giving these systems the kind of edge needed to keep pace with evolving fraud techniques. Understanding these advantages not only informs stakeholders but also sets the stage for continuous innovation and improvement.
Increased Accuracy in Identification
At the core of any effective fraud detection system lies its accuracy. Traditional methods often fall short due to their reliance on historical data and predictable patterns. In contrast, AI systems leverage machine learning algorithms that adapt and learn from new data. This enhances their ability to discern legitimate transactions from fraudulent ones, reducing false positives that can plague conventional systems.
Consider a scenario where a crypto exchange detects an unusual withdrawal pattern. Traditional systems might flag several genuine activities as suspicious, resulting in customer frustration. AI, however, analyzes multiple variables in real-time, including transaction history, user behavior, and global market trends, to provide a more nuanced understanding of potential fraud risks. Hence, organizations could see a marked improvement in their fraud detection ratios, preserving customer trust and ensuring smoother operations.
Real-Time Monitoring Capabilities
Speed is another crucial element that distinguishes AI-led fraud detection from traditional methods. In a world where cryptocurrencies are traded around the clock, the need for real-time monitoring is paramount. AI excels in this area by providing instant alerts and responses to suspicious activities.
Through continuous surveillance of transactions, AI can quickly identify anomalies that might indicate fraudulent intent. This capability is akin to having a highly vigilant security guard monitoring the exchanges 24/7, catching any potential threats before they escalate. For instance, if an account suddenly starts executing large trades from an unusual location, the AI system can flag this behavior immediately and trigger a verification process, potentially stopping fraud in its tracks.
Real-time monitoring allows companies not just to react to fraud but to preempt it. This proactive approach can significantly increase the organization’s security posture in an ever-changing threat landscape.
In summary, the benefits of AI-led fraud detection in the cryptocurrency sector cannot be overstated. By ensuring heightened accuracy in identifying fraudulent activities and enabling real-time monitoring, AI tools are reshaping the way businesses protect themselves and their clients against fraud. As technology continues to evolve, so should our strategies in mitigating risks, making AI an indispensable ally in the fight against cryptocurrency fraud.
Challenges in Adopting AI Solutions
Adopting artificial intelligence in fraud detection within the cryptocurrency landscape is a step many organizations contemplate. However, this journey is far from simple. Various obstacles stand in the way, making it crucial for stakeholders to understand these challenges thoroughly.
Data Privacy Concerns
One of the foremost challenges resonates around data privacy. The nature of cryptocurrencies inherently involves a multitude of transactions with sensitive information. When developing AI solutions, organizations must navigate the complexities of data protection laws, such as the EU's General Data Protection Regulation (GDPR). The essence of these regulations is to safeguard personal data while utilizing it for analysis and detection.
Incorporating AI requires vast amounts of data for training algorithms; however, striking a balance between effective fraud detection and adhering to legal frameworks often becomes a daunting task. As organizations move to collect transaction data, ensuring that they do not infringe on users' privacy rights is paramount. This means investing in privacy-preserving technologies must not be overlooked.
Some techniques include:
- Anonymization of data to maintain user confidentiality
- Utilizing federated learning whereby the AI model is trained across many decentralized devices while keeping data local
- Implementing strong encryption methods protecting data storage and transmission.
These approaches can serve as a hedge against potential privacy violations while still enabling robust detection models.
"The process of aligning AI capabilities with privacy requirements may deter potential investors, but those who can navigate these waters effectively will hold a significant advantage."
Scalability Issues
Another layer of complexity arises from scalability issues. As the cryptocurrency market grows, so too does the volume of transactions. AI systems must be able to adapt and expand to handle this increasing scale without compromising on performance.
Many existing fraud detection systems simply weren’t built with scalability in mind. When transaction volumes surge, traditional systems may struggle, leading to slower response times or increased false positives, which can undermine the very purpose of implementing AI.
Scaling AI systems involves addressing several factors:
- Infrastructure robustness: Businesses must ensure their hardware and software can handle increased data flows without crashing.
- Choosing cloud-based solutions offers flexibility and enhances capacity, enabling real-time processing of vast amounts of data.
- Continuous model retraining is needed to adapt to new fraudulent techniques, therefore a seamless integration process into current systems is necessary.
As organizations face these scalability hurdles, they must consider investing in technology that can grow alongside their operations.
Understanding and addressing these challenges is essential not only for the successful deployment of AI solutions but also for building trust with users in an already volatile environment like cryptocurrency.
By proactively tackling data privacy concerns and scalability issues, entities can unlock the potential of AI in enhancing fraud detection while ensuring compliance and efficiency.
Comparing AI with Traditional Fraud Detection Methods
Understanding the contrast between artificial intelligence and traditional methods of fraud detection is crucial for those interested in the cryptocurrency realm. Each method boasts its own unique approach to combating fraudulent activity, and recognizing their differences can help stakeholders make informed decisions. Most importantly, this discussion sheds light on how AI elevates the standards of fraud detection beyond the capabilities of older systems.
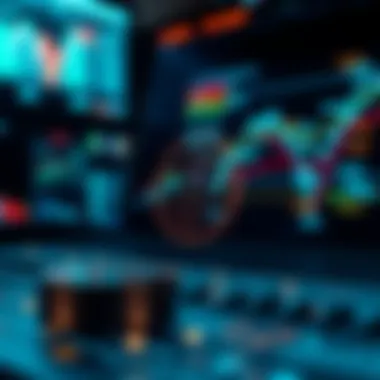
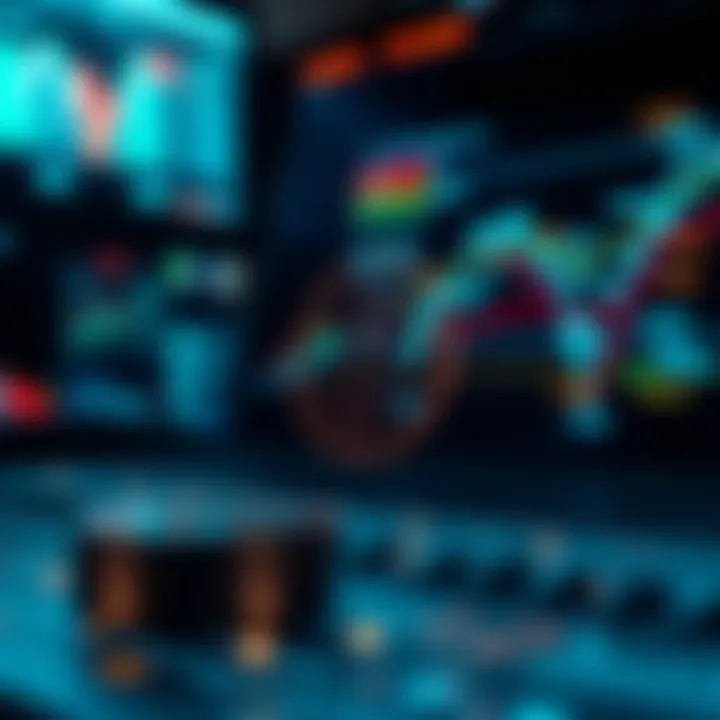
Efficacy of Traditional Systems
Traditional fraud detection systems have long been the backbone of financial security mechanisms. They typically rely on rule-based algorithms, which examine transactions against pre-defined criteria. For example, if a transaction amount exceeds a certain threshold or originates from a flagged location, the system raises an alert. While this approach has historically served industries, its limitations surface distinctly in the fast-paced world of cryptocurrency.
The efficacy of traditional systems in detecting fraud pivots on several factors:
- Latency: Traditional systems often analyze data in batches, leading to delayed responses. In crypto, where speeds are key, this can create delays that cost organizations dearly.
- Adaptability: Fraud tactics evolve quickly, and traditional systems struggle to keep up. Static rules don't adjust easily, which means new scams could slip through unnoticed.
- Volume of Data: The sheer amount of transactions conducted in cryptocurrency contexts can overwhelm these outdated systems. They may miss subtle indicators of fraud that AI-driven systems could easily spot.
Advantages of AI Approaches
AI fraud detection offers a modern, robust alternative greater than its traditional counterparts. With the diversification of fraud techniques, AI shines through several significant advantages:
- Real-Time Risk Assessment: AI can study transactions as they happen, analyzing data streams instantaneously. It identifies potentially fraudulent behavior and triggers alerts immediately, reducing response time dramatically.
- Learning from Past Events: Unlike static, rule-based systems, AI employs machine learning. This allows it to learn from historical data. For instance, if a certain pattern of behavior usually leads to fraud, an AI model adapts to recognize similar patterns in future transactions.
- Behavioral Analytics: AI can evaluate users' behavior in-depth, employing artificial intelligence techniques to create user profiles. This helps in identifying anomalies that may flag fraudulent activities effectively. For example, if a user typically transacts small amounts and suddenly attempts a large withdrawal, AI can deem this suspicious and react accordingly.
- Scalability: AI systems can scale more efficiently to handle the exponential growth crowds seen within cryptocurrencies. They can process vast amounts of data without a significant drop in performance, making them ideal for the increasing transaction volumes seen in the crypto space.
As organizations invest in AI technologies, they not only bolster their defense mechanisms against fraud but also adapt to the rapidly changing landscape of cryptocurrency.
In short, AI brings a level of dynamism and depth to fraud detection methods, effectively addressing the weaknesses inherent in traditional systems.
This exploration is vital for investors, developers, and stakeholders who seek to understand how to remain ahead in the fight against cryptocurrency fraud. The next phase should be a closer look at ethical considerations in implementing these advanced systems.
Case Studies of Successful AI Implementation
In the rapidly evolving landscape of cryptocurrency, AI-driven fraud detection systems have shown significant promise. The real-world application of these technologies offers a goldmine of insights into not only their efficacy but also the practical challenges they surmount. This section highlights key case studies that showcase successful implementations of AI in the field of fraud detection.
Industry Leaders Leveraging AI
Leading companies in the cryptocurrency sector are not blind to the potential of AI. Pioneers like Chainalysis and Elliptic have implemented sophisticated AI algorithms to combat fraud. By employing machine learning techniques, these companies have developed models capable of identifying fraudulent patterns in transactions and even predicting future risks.
- Chainalysis uses AI to analyze vast datasets derived from blockchain activity, offering real-time alerts for suspicious transactions. Their algorithms can differentiate between legitimate transactions and those potentially linked to criminal activities, thus enhancing overall security.
- Elliptic, on the other hand, employs a combination of AI and expert human analysis to vet transactions. They provide clients with tools that highlight suspicious patterns, giving organizations the ability to act before significant losses accrue.
These examples reflect a shift from traditional static detection methods to more robust, AI-enhanced approaches. The companies not only streamline their operations but also provide a safer environment for users, effectively reducing the risk and impact of fraudulent activities.
Analysis of Impact on Fraud Rates
The impact of AI on fraud detection isn’t just theoretical—numerous studies and reports indicate a tangible reduction in fraud rates following the implementation of AI systems. In fact, many organizations have reported sharp drops in successful fraud attempts. For instance, a case study by the Cryptocurrency Security Organization found that businesses leveraging AI tools experienced up to a 30% decline in fraud incidents over a year.
In terms of quantifying success:
- Transaction Analysis: With AI's predictive analytics, organizations can assess transaction anomalies quickly, mitigating risks before they escalate.
- User Trust: The implementation of AI solutions has fostered an increased sense of security among users. In survey findings, businesses that adopted AI reported higher consumer confidence, which directly correlates with increased engagement.
- Continuous Improvement: The iterative nature of AI systems allows for ongoing learning from fraud patterns, which ultimately refines detection mechanisms further.
"Integrating AI into fraud detection isn’t just about technology, it’s about reshaping how businesses perceive and react to threats." This shift not only aids in catching fraud as it happens but cultivates a proactive culture of vigilance against potential threats.
Future Trends in AI and Fraud Detection
The landscape of fraud detection in cryptocurrency is evolving at a blistering pace. As technology marches on, so does the cunning of fraudsters; thus, staying ahead of the game requires a proactive approach to fraud management. This section explores emerging trends that signify a shift in how AI systems can enhance security and refine detection methods. The ultimate goal is not simply to keep up with fraudulent techniques but to outsmart them.
Integrated AI Models
Integrated AI models represent a significant advancement in the realm of fraud detection. By combining various artificial intelligence techniques, such as machine learning, natural language processing, and neural networks, these models create a comprehensive framework capable of identifying suspicious behavior across multiple vectors.
For instance, consider a scenario where a platform utilizes integrated models where data on transaction patterns is analyzed alongside social media activity. This dual-layered scrutiny can flag red flags that might go unnoticed if examining only one source. The marriage of diverse AI techniques allows for more robust and resilient fraud detection frameworks.
Some key aspects of integrated AI models include:
- Holistic Analysis: By pulling data from various sources, these models can recognize patterns that indicate potential fraud more reliably.
- Adaptability: Integrated models can quickly adapt to new types of fraud by learning from a broader array of inputs.
- Enhanced Accuracy: With multiple data streams, the likelihood of false positives can decrease, allowing for a more streamlined approach to legitimate transactions.
Implementing such models often requires significant computational resources, but the payoff can be enormous. Improving the Early Warning Systems (EWS) through integrated AI models can allow crypto exchanges and wallets to act swiftly and prevent larger-scale incursions.
Collaboration Across the Industry
The ever-growing challenge of fraud within the cryptocurrency sector necessitates a collaborative approach. No single entity can combat fraud in isolation, especially when the talent pool is spread thin across numerous platforms and users worldwide. Cooperation between stakeholders—such as exchanges, law enforcement, and regulatory bodies—can yield a powerful united front against potential threats.
Collaboration across the industry brings forth several advantages:
- Knowledge Sharing: Organizations can share insights and best practices. This can include sharing information on recent fraud trends or tactics that have been effective in stopping scams.
- Collective Defense Strategies: Working together enables the creation of collective strategies that share the burden of infrastructure investment. This approach allows smaller firms that may not have the capital for advanced technologies to participate in wider network defenses.
- Standardization of Protocols: Industry-wide collaboration can also lead to the establishment of unified standards for security. This can help in ensuring everyone is on the same page when it comes to recognizing and addressing fraud threats.
Ethical Considerations in AI Usage
As artificial intelligence continues to weave its intricate web across various domains, its role in fraud detection within cryptocurrency is becoming increasingly significant. This section aims to probe deep into the ethical considerations surrounding the use of AI in this context. Understanding these nuances is not merely academic; it has practical implications for investors, enthusiasts, and regulators alike. Ethical AI practices can shape the future landscape of financial technologies, emphasizing responsible usage that ensures trust and safety in digital transactions.
Responsible AI Practices
Responsible AI practices are the linchpins in ensuring that technology serves its intended purpose without compromising ethical standards. These practices encompass several dimensions:
- Bias Mitigation: AI models are only as good as the data fed to them. If data reflects societal biases, the model’s decisions may inadvertently perpetuate these biases. Therefore, rigorous testing and refinement of algorithms are crucial to ensuring fairness across different demographics.
- User Privacy: In an age where data is often more valuable than gold, safeguarding user privacy is imperative. Implementing policies that ensure personal data is anonymized and protected can sustain user trust and avoid legal pitfalls.
- Security Measures: Beyond just fraud detection, AI systems themselves need robust security frameworks to prevent hijacking or manipulation. An ethical AI approach mandates continual assessments of security protocols.
To encapsulate, when these responsible practices are rigorously followed, they not only enhance the efficacy of fraud detection systems but also instill confidence among users and stakeholders.
Transparency and Accountability
Transparency in AI is often seen as a noble goal yet is frequently elusive in practice. For AI systems to be embraced within the cryptocurrency realm, it is vital to strike a balance between sophisticated algorithms and user understanding.
To ensure transparency:
- Explainable AI: This emphasizes that model decisions should be interpretable by users. When algorithms make conclusions regarding fraudulent activities, stakeholders should understand the basis of these decisions, fostering trust.
- Audit Trails: Regular auditing of AI systems can ensure that they behave as expected and are accountable. This should involve third-party evaluations to validate processes, which can bolster industry credibility.
In this digital age, the crux of transparency isn’t just about ensuring systems work; it's about proving they work fairly and ethically. Only then can the full potential of AI in fraud detection be realized, safeguarding the interests of all participants in the crypto ecosystem.
"To navigate the complexities of AI in fraud detection, we must forego opacity in favor of clarity, ensuring accountability at every turn."
In summary, the ethical considerations in implementing AI innovations are fundamental in paving the way for a more secure financial future. By adhering to responsible practices, ensuring transparency and instituting accountability, stakeholders can foster an environment ripe for innovation while mitigating ethical risks. The integration of these elements not only enhances trust in AI systems but also fortifies the overall integrity of the cryptocurrency landscape.
Regulatory Implications for AI in Fraud Detection
The regulatory landscape surrounding artificial intelligence in fraud detection is a necessary component that directs the course of this technology. AI’s presence in cryptocurrency fraud detection is not just about efficiency and accuracy; it also straddles a complex web of regulations that shape its implementation. The importance of understanding these implications can’t be overstated, especially given the rapid evolution of both the technology and the regulatory frameworks designed to oversee them.
Without clear guidelines, companies might fall into the trap of making decisions that aren't just inefficient; they could also be illegal. Compliance is crucial not only for mitigating risks but also for ensuring that stakeholders feel secure about their investments in AI technologies.
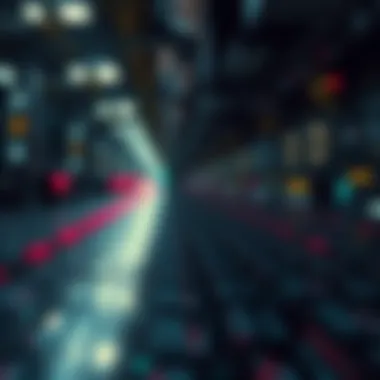
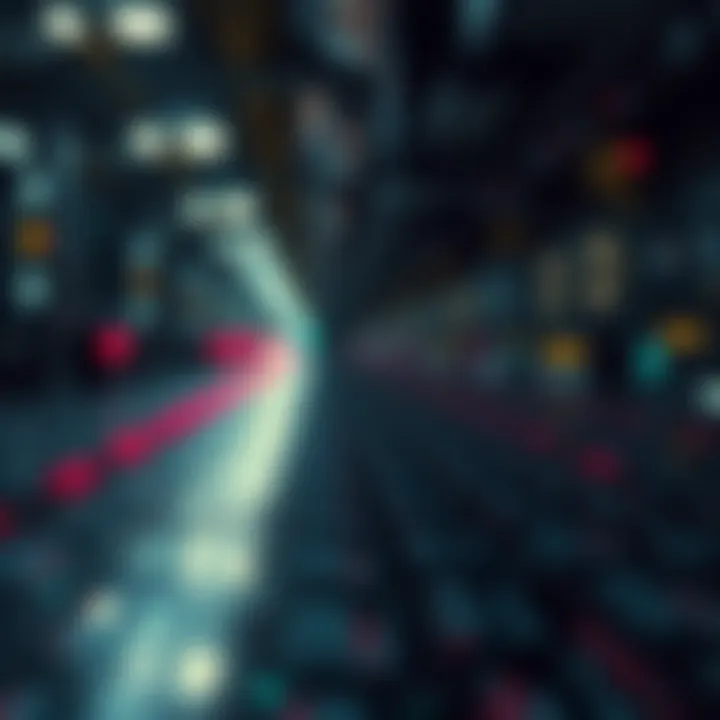
The journey towards effective regulatory frameworks involves several factors:
- Informed Policy Making: Regulators need insights that stem from both technological understanding and the realities of the crypto space.
- Risk Mitigation: With fraud being a persistent threat, regulations must ensure that the application of AI in this domain doesn’t open new avenues for exploitation.
- Stakeholder Trust: Establishing clear guidelines allows investors and users to have confidence in the systems implemented.
In essence, regulatory implications shape the environment in which AI technologies operate and directly influence the efficacy of fraud detection systems.
Current Regulations and Compliance
Navigating the current regulatory environment requires attention to a patchwork of laws that vary significantly from one jurisdiction to another. For example, countries like the United States have specific regulations under the Securities and Exchange Commission (SEC), while European nations may adhere to standards set forth by the General Data Protection Regulation (GDPR). Here are key considerations:
- Data Privacy: Regulations such as GDPR impose stringent guidelines on how personal data can be used in AI models, which necessitates transparent data practices. If companies use AI without proper data governance, they could face heavy fines.
- Financial Transparency: Different financial regulations require entities to disclose AI usage in fraud detection. This includes explaining how AI contributes to their compliance and operational strategies.
- Licensing and Oversight: Many jurisdictions are exploring if and how companies deploying AI in the financial sector should be licensed, ensuring that these technologies are subject to a level of oversight akin to traditional financial systems.
"Regulatory frameworks are not just red tape; they are essential scaffolding that supports innovation while protecting the interests of users."
Future Regulatory Considerations
Looking to the future, regulatory considerations will evolve as technology progresses. Here are a few potential directions:
- AI Accountability: Future regulations might mandate that AI systems must be interpretable, offering insights into how decisions regarding fraud detection are made. This could involve having a human review component or explainable AI.
- Collaborative Frameworks: There’s a growing call for global cooperation among regulators to create cohesive standards that accommodate the borderless nature of cryptocurrencies. An internationally recognized framework could help streamline compliance and enhance the efficacy of fraud detection worldwide.
- Dynamic Regulations: Regulations may need to be adaptable to keep pace with technological advancements. This will ensure that outdated rules do not hinder innovation while continuing to protect against risks efficiently.
Ensuring that regulations evolve in step with technology will be crucial for balancing efficiency, security, and user trust in AI's application across the cryptocurrency landscape.
Insights from Experts in the Field
The conversation around AI's role in fraud detection within cryptocurrency is continuously evolving. With the pace at which both technologies and illicit behaviors adapt, it is crucial to tap into insights from those who are deep within the trenches—experts in data science and regulatory authorities. This section aims to provide a nuanced understanding of both the technical advancements that AI brings to the table and the regulatory frameworks that shape its usage.
Interviews with Data Scientists
A good start is listening to data scientists who have dedicated years to exploring machine learning and its application in detecting fraud. One key insight from recent interviews with professionals in this area is the significant transformation brought about by algorithms that can learn from patterns. They emphasize that the beauty of AI lies in its ability to not just recognize known fraudulent activities, but also to identify anomalies that the human eye might miss. For example, a data scientist might point out how unsupervised learning techniques can flag transactions that deviate from typical behaviors, even if they have not been categorized as fraud in the past.
- Adaptive Learning: One expert explained that their system utilizes reinforcement learning, allowing the AI to adjust its parameters in real-time. This adaptability is crucial in the crypto landscape, where fraud tactics can change overnight, leaving traditional methods in the dust.
- Real-World Applications: Through detailed case studies, interviews reveal that firms using AI have seen reductions in false positives by as much as 30%, allowing genuine transactions to go through while effectively catching nefarious ones. It’s a delicate dance, balancing security with user experience.
- Collaboration Tools: The role of collaboration among data scientists cannot be understated. They share methodologies and findings with one another, often leading to innovations that each may not have conceived individually. This sharing of ideas has led to the development of robust frameworks that standardize how AI models are evaluated.
Perspectives from Regulatory Authorities
Now consider the perspective of regulatory authorities, who play a pivotal role in ensuring that AI technologies comply with existing laws. Their stance often provides a juxtaposition to that of data scientists. These authorities argue that while AI possesses immense potential, it is not without consequences. They emphasize several key points:
- Compliance Requirements: Ensuring that AI systems adhere to anti-money laundering (AML) and know-your-customer (KYC) guidelines is paramount. Regulatory experts assert that as firms incorporate AI into their systems, they must also establish protocols for accountability and transparency.
- Balancing Innovation with Oversight: Several regulatory officials have voiced the need for balance. They understand that innovation can sometimes outpace regulations, leading to grey areas. There’s an ongoing dialogue about crafting frameworks that encourage growth while still protecting consumers.
- Public Trust: Another critical point made by authorities is the necessity for public trust in AI systems. As they articulate, if users feel that AI is a black box without transparency, widespread adoption could falter. Regular communications and transparency into how AI systems operate can help significantly.
"Navigating the landscape of cryptocurrency fraud detection with AI necessitates a dance between innovation, regulation, and most importantly, ethical considerations," said one regulatory expert.
In summary, integrating insights from both data scientists and regulatory authorities fosters a more rounded understanding of how AI can reshape fraud detection in cryptocurrency. By weaving together technological advancements with regulatory awareness, a more secure and effective framework can emerge, benefiting both users and industry stakeholders alike.
Best Practices for Implementing AI in Fraud Detection
To harness the full potential of AI in detecting and preventing fraud in the cryptocurrency realm, organizations must follow certain best practices. Implementing AI solutions without a robust strategy can lead to issues such as ineffective algorithms, wasted resources, and missed fraud attempts. By adhering to structured approaches, companies can optimize their fraud detection capabilities and adjust to the dynamic nature of threats.
Creating a Robust Framework
Building a solid framework for implementing AI in fraud detection is paramount. This framework should encompass a combination of technological infrastructure, data management processes, and clearly defined goals. To achieve this, organizations should consider the following points:
- Defining Objectives: It’s crucial to understand what specific types of fraud are most prevalent, whether it’s phishing scams, Ponzi schemes, or unauthorized transactions. Clear objectives help in tailoring AI models to efficiently address these threats.
- Data Management: Effective data governance cannot be overlooked. Organizations must ensure their datasets are not only vast but also of high quality to allow machine learning models to learn accurately. Data should be cleaned, categorized, and updated regularly to reflect current trends in fraud.
- Choosing the Right Tools: With many AI tools available, selecting one that aligns with organizational needs and resources is critical. Solutions should be scalable and flexible enough to adapt as fraud patterns evolve. For example, TensorFlow or PyTorch can be employed to build customized predictive models.
- Interdisciplinary Teams: Combining expertise from different fields, such as finance, data science, and cybersecurity, can lead to innovative approaches in detecting fraud. Team members should exchange knowledge and collaborate frequently.
Building this robust framework lays the groundwork for effective AI implementation, ensuring that organizations are not just reactive, but proactive in combating fraud.
Continuous Evaluation and Improvement
Once AI systems have been implemented, a continuous evaluation process is essential. The landscape of cryptocurrency fraud is ever-changing, and what worked yesterday may not work today. Continuous improvement requires a multi-faceted approach that includes:
- Performance Monitoring: Regularly measuring the performance of AI models is key to ensuring they remain effective. Metrics such as false positive rates, detection speed, and overall accuracy should be monitored closely. If models begin to underperform, investigations should determine the cause.
- Feedback Loops: Establishing feedback mechanisms allows teams to gather insights from stakeholders, including fraud analysts and end-users. These can reveal blind spots and potential areas for improvement in the AI systems.
- Staying Informed: The cryptocurrency market is known for its rapid evolution. It’s important for teams to be aware of new fraud trends, technological advancements, and regulatory changes. This might involve participating in industry workshops, forums, or subscribing to relevant publications.
- Iterative Testing: Applying an agile methodology to AI model development can ensure that solutions are constantly refined. Running pilot tests on new algorithms can help gauge their effectiveness before full integration.
Incorporating these continuous evaluation and improvement strategies can significantly enhance the longevity and effectiveness of AI-driven fraud detection systems in a rapidly changing environment.
Important Note: Staying ahead in the game involves both the evolution of technology and a keen understanding of the fraud landscape. AI must be seen not just as a tool, but as an integral component of a broader security strategy.
By following these best practices, organizations can fortify their defenses against fraud while leveraging the advanced capabilities offered by AI.
Endings on AI’s Impact on the Crypto Landscape
The incorporation of artificial intelligence into the realm of cryptocurrency fraud detection marks a significant milestone in combating financial malpractice. Given the decentralized and largely unregulated nature of cryptocurrencies, the potential for fraud is amplified, making effective detection systems indispensable. AI's role cannot be overstated; it not only helps in identifying fraudulent activities faster but also enhances the accuracy of these identifications. This is critical, especially when you consider the rapid pace at which fraud techniques evolve, often outpacing traditional detection methods.
In this section, we summarize the essential takeaways while looking toward the future of fraud detection in the crypto world.
Summarizing Key Takeaways
- Enhancement of Detection Rates: AI’s machine learning capabilities allow systems to learn from historical data, making them increasingly proficient in recognizing patterns of fraudulent behavior. This results in a notable boost in detection rates compared to traditional systems.
- Real-Time Response: With AI, organizations can monitor transactions and activities in real time. This immediacy in response reduces the window for fraudsters to execute their schemes before any detection occurs.
- Dynamic Learning Algorithms: Unlike static rules used in conventional systems, AI algorithms adapt over time. They analyze new data continually, ensuring that the detection methods remain effective against new fraud tactics.
- Cost Efficiency: Implementing AI-driven systems can significantly decrease operational costs associated with fraud detection over time. Though initial investments may be high, the long-term savings and prevention of financial losses make it worthwhile.
- Stakeholder Confidence: Enhanced fraud detection not only protects the financial institutions but also instills trust among investors and users in the cryptocurrency space. Confidence is crucial in encouraging broader adoption of digital currencies.
Looking Ahead: The Future of Fraud Detection
The future of fraud detection in cryptocurrency, propelled by AI advancements, seems promising but comes with its set of challenges. Emerging trends include:
- Integration Across Platforms: We expect to see AI models that integrate with various systems, creating a seamless fraud detection ecosystem. This means that a transaction flagged in one platform could alert others across the network, increasing safety.
- Collaborative Approaches: The industry may trend towards cooperative frameworks where competitors share anonymized data to refine detection techniques further. This collaboration could allow for a more holistic view of fraudulent activities.
- Ethical AI Practices: As AI becomes more embedded in fraud detection, the ethical implications of its use become increasingly important. Ensuring transparency in algorithms and maintaining data privacy should be paramount.
- Regulatory Adjustments: As technology evolves, so too must regulations surrounding it. Future regulations will need to address the nuances of AI in finance, suggesting proactive compliance measures for organizations.
Further Reading and Resources
Understanding the landscape of AI in cryptocurrency fraud detection requires more than just a glimpse or a surface-level glance. For those interested in engaging deeply with this topic, further reading and resources are vital to gaining a robust comprehension of the methodologies and technologies involved. These resources serve as signposts that guide you through the complexities, presenting invaluable insights into both the theoretical frameworks and practical applications of AI in identifying fraudulent activities. Not only do they provide a broader context, but they also help in aligning one's own practices with the latest developments in the field.
Books on AI and Fraud Prevention
Unquestionably, literature can be a powerful vehicle for enhancing one's understanding of intricate subjects like AI in fraud detection. Books often present structured insights, allowing readers to grasp concepts at their own pace. Some notable titles include:
- "Artificial Intelligence in Financial Services" by S. J. Tracton
This book dives into how AI and machine learning reshape the financial landscape, including fraud detection mechanisms. - "Fraud Analytics Using Descriptive, Predictive, and Social Network Techniques" by Daniel P. O'Leary
It offers a comprehensive overview of the data analytics involved in identifying fraud patterns, which is crucial for developing AI solutions. - "Data Mining for Business Intelligence" by Galit Shmueli and Nitin R. Patel
Although it covers a broader spectrum, this book equips readers with techniques that are directly applicable to fraud detection in cryptocurrencies.
Many of these works are not just theoretical; they provide practical case studies and examples that show how AI can be leveraged to create more secure environments within the cryptocurrency sphere. Engaging with such texts allows investors, developers, and analysts to frame their strategies in a well-informed manner.
Research Papers and Journals
Research papers are another cornerstone for anyone looking to understand the AI landscape in fraud detection more profoundly. Academic journals publish cutting-edge studies that explore both the latest techniques and the conceptual underpinnings of AI applications in financial security. For a more in-depth exploration, consider the following:
- "A Survey on Fraud Detection System in Banking Transactions"
This research paper discusses various methods, including AI, and assesses their efficacy in identifying fraudulent transactions across diverse banking contexts. - "Decentralized Blockchain Security: A Review and Future Directions"
Exploring fraud detection's intersection with blockchain technology, this paper offers insights on safeguarding transactions in cryptocurrency ecosystems. - "Machine Learning for Financial Fraud Detection: Techniques and Applications"
This scholarly article presents a thorough review of machine learning methods specifically tailored for combating fraud, making a significant contribution to understanding the implementation nuances.
In short, diving into research papers brings a wealth of empirical evidence and rigorous analysis that textbook knowledge might lack. This allows readers to stay not just informed but ahead in their understanding of how AI can dynamically shift in response to evolving fraudulent tactics in cryptocurrency.
The role of continuous education through reading can’t be overstated. Knowledge accumulates, leading to a more nuanced understanding and sharper detection capabilities.